Put simply, generative AI refers to algorithms capable of producing content across various formats, such as text, images, videos, simulations, code, and audio.
Generative AI typically involves using deep learning algorithms, like generative adversarial networks (GANs) and variational auto-encoders (VAEs), that are trained on large datasets of existing content. These algorithms can then generate new content by extrapolating from the patterns and features of the training data.

How does generative AI work?
Generative AI uses a model to learn a dataset’s underlying patterns and structure without being explicitly told what to look for. This approach differs from predictive AI, which uses labeled data to make predictions.
Generative AI models can be trained on various data types, such as text, images, or audio. The goal of the model is to learn the underlying probability distribution of the data, which allows it to generate new data similar to the original dataset.

What are ChatGPT, DALL-E, and other examples of AI applications?
ChatGPT is a language model pre-trained on vast amounts of text data, allowing it to generate real-time human-like responses to user queries.
ChatGPT uses pre-trained knowledge to generate a response to the user’s question that is relevant to the input. Depending on the context, this can vary in length, style, and tone. The application also uses machine learning algorithms to continuously improve its responses based on user feedback. This means that as more people use and interact with ChatGPT, it becomes better at generating relevant and accurate answers.
Like ChatGPT, DALLE-2 is also developed by OpenAI, an AI research and deployment company. It combines deep learning and NLP to create images from textual descriptions. It is trained on a large dataset of images and text, which enables it to learn how to generate ideas that accurately match the corresponding textual input.
Although these are two of the most famous examples, many AI apps are available, with new ones constantly being developed, such as HuggingChat, an open-source alternative to ChatGPT.
What companies are using generative AI?
Big tech companies like Amazon, Microsoft, and Google have all integrated AI into their products.

Arguably, one of the most famous examples of AI in recent times is Amazon’s Alexa and Siri voice assistants. At the same time, Microsoft Bing has introduced OpenAI features to its search engine, including its very own AI art generator powered by DALL-E called Microsoft Image Creator. Google has responded by creating ChatGPT competitor Bard and is working on a new version of its search engine called Project Magi.
Other companies integrating AI into their products include Notion, Buffer, Quora, and Grammarly.
What is the difference between predictive AI and generative AI?
Predictive AI (supervised learning) involves training a model on labeled data to predict new, unseen data. This approach relies on historical data to predict future events or outcomes. Predictive AI aims to make accurate predictions based on a set of features or inputs.
Generative AI, on the other hand, involves creating new data that is similar to a given dataset. This approach generates new content, such as images, videos, or text.

What’s the difference between AI (artificial intelligence) and ML (machine learning)?
Artificial intelligence involves creating machines that perform tasks that need human intelligence, such as perception, reasoning, learning, problem-solving, and decision-making. It encompasses various techniques and approaches, including expert systems, rule-based systems, and neural networks.
Artificial Intelligence is a broad field, and Machine Learning is a specific subset that aims to improve the performance of algorithms by training them to learn from data without being explicitly programmed. In essence, Machine Learning is a means of achieving AI.
Why is generative AI so popular?
The ability to create new content, the flexibility of unsupervised learning, advances in deep understanding, the availability of large datasets, and a wide range of applications have all made generative AI a popular area of research and development.
Generative AI has numerous applications across industries, including entertainment, gaming, marketing, and healthcare. It can create personalized content for marketing campaigns, generate realistic simulations for training purposes, or produce synthetic data for medical research.
What are the dangers of generative AI?
There are several concerns around generative AI, including ethical and privacy concerns. AI art generators, for example, have raised issues around artist copyright: how the tools are trained and who owns the content generated by these models.
The spread of misinformation and fake content has also generated news headlines recently, with counterfeit images of Donald Trump and The Pope going viral, leading to questions about how to spot an AI-generated image.
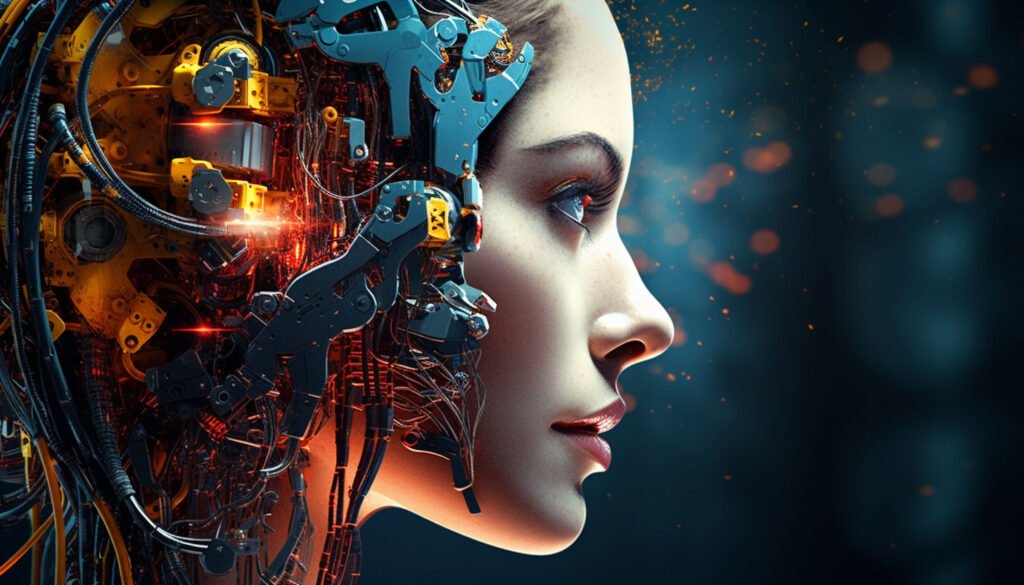
Privacy and data protection has also been frequently cited as a danger of generative AI tools. ChatGPT has been banned in several countries, including Italy, after the country’s data protection watchdog claimed that ChatGPT did not comply with the European General Data Protection Regulation (GDPR).
Privacy concerns have been thrust into the spotlight as generative AI models can be trained on personal data, such as images or voice recordings. OpenAI is introducing new ways to turn off chat history, allowing users to decide which conversations can be used to train their models.